Arijit Mukherjee decodes Netflix’s cutting-edge data science techniques
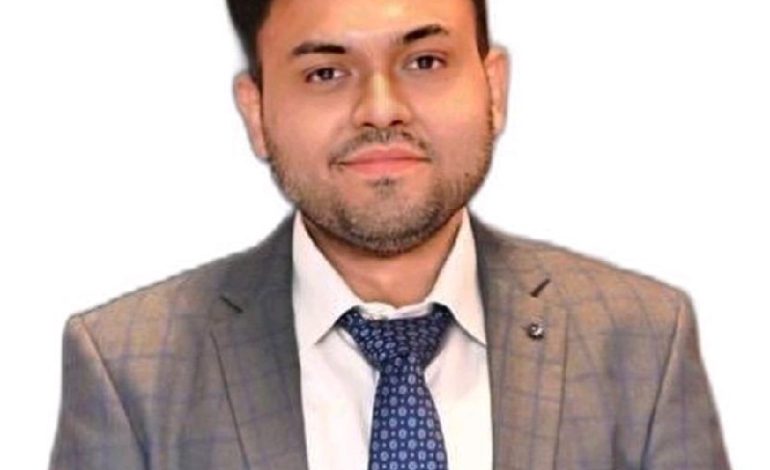
Do you ever find yourself amazed by how accurately Netflix suggests shows and movies you’ll love? It’s not magic; it’s data science! Recently, Arijit Mukherjee, a renowned data science consultant at Accenture, conducted a mind-blowing webinar titled “Decoding the Binge Effect: How does NETFLIX Build a Recommendation Engine.” This groundbreaking webinar, hosted by ATLAS SkillTech University, not only delved into Netflix’s cutting-edge data science techniques but also introduced the university’s prestigious B.Tech program in Computer Science, Artificial Intelligence and Machine Learning. For participants, it was an incredible opportunity to explore the realm of intelligent systems and embark on the path to becoming the future AI masterminds.
Why does Netflix need a recommendation system?
Netflix’s recommendation system serves multiple purposes, and during the webinar, Arijit highlighted two key reasons:
Revenue
By suggesting content tailored to users’ preferences, Netflix can increase customer satisfaction and engagement, leading to higher retention rates. This translates into substantial savings on customer acquisition costs.
The Binge Effect
Netflix understands the power of binge-watching and aims to keep viewers hooked. The recommendation system plays a vital role in suggesting relevant and captivating content, encouraging users to continue their binge-watching experience.
How does Netflix rank titles?
Netflix employs a unique ranking system for its vast catalog of titles. During the webinar, Arijit shed light on this process:
Within each row
Titles are ranked within each row, with the strongest recommendations appearing on the left side. This arrangement allows users to quickly identify appealing options.
Across rows
Each row highlights a specific theme, such as “Top 10,” “Trending,” or a particular genre. One algorithm generates each row, ensuring a cohesive selection of titles that align with users’ preferences.
Why Rows?
Rows present a coherent and visually appealing way to display similar items. Users can easily scroll through rows and determine their interest in a particular category. From Netflix’s perspective, rows also provide valuable feedback; a right-scroll indicates interest, while a scroll-down suggests non-interest.
Meet the Algorithms
During the webinar, Arijit introduced several algorithms employed by Netflix’s recommendation system. Let’s explore a few of them:
Cinematch
Netflix’s primary algorithm, Cinematch, uses item-based collaborative filtering. It identifies similarities between movies and shows based on user ratings and behaviors, then makes recommendations based on the preferences of similar users and their ratings of similar items. Cinematch continually learns from user feedback, adapting and refining its recommendations over time.
Video-Video Similarity Ranker
This ranker utilises an item-item similarity matrix to compute similarities between consumed items and other available items. By leveraging similarity scores, the algorithm recommends the most similar items to the user. It’s unpersonalised in the sense that it doesn’t rely on user-specific information, but personalised in displaying similar items on a member’s homepage.
How does Netflix filter by content?
Netflix’s recommendation system leverages various techniques to filter content effectively. Arijit discussed two important aspects:
Similarity Matrix
A live example demonstrated the power of a similarity matrix. By analysing content similarities, Netflix can generate recommendations based on users’ preferences and viewing patterns.
Page Generation
The row generation process, as illustrated during the webinar, showcases how algorithms generate candidate rows. Netflix employs a Machine Learning approach, mean average precision at M by N, to select which rows to display from thousands of options.
Advantages of a recommendation system
Arijit highlighted the advantages of Netflix’s recommendation system:
Product category discovery
The system assists users in discovering new genres and expanding their viewing horizons.
Report Generation
By analysing user interactions and preferences, Netflix generates reports that provide valuable insights into viewer behavior.
Customer Satisfaction
Personalised recommendations enhance user satisfaction by presenting content tailored to individual tastes and preferences.
Netflix’s recommendation system revolutionises the way we discover and enjoy TV shows and movies. Through advanced algorithms, personalised recommendations and continual improvement, Netflix enhances the user experience, increases engagement and promotes customer retention.
The webinar conducted by Arijit Mukherjee served as a gateway to understanding the power of data science and its application in the field of Artificial Intelligence and Machine Learning. To unlock a whole new world of intelligent systems and know more about the ATLAS B.Tech program in Computer Science, AI & ML, visit: https://atlasuniversity.edu.in/ugdx/